The rsRNA:target gene interactions were identified primarily using TAREF and Targetscan, which were validated using experimental data from Argonaute CLIP-sequencing (14 experimental conditions), CLASH-sequencing(1 experiment), anti-coexpression for expression using protein abundance data (4776 individuals) and RNA expression data from s/RNA-seq and microarrays studies (3013 conditions). From these analyses we have identified several important genes involved in the regulation of cancer, pathways related to cancer, growth and development, cell cycle and apoptosis are highly represented. The analyses performed in this study suggest that these rsRNAs originate from multiple loci including repetitive elements and intronic regions. Also these novel rsRNAs share highly conserved seed region with known mature miRNAs of humans reported in miRBase version 21. Furthermore, these rsRNAs were searched acrossed the sRNAs expressed specifically in the presence of DGCR8 and which were absent in the DGCR8 knockout in order to identify rsRNAs processed by DGCR8 microprocessor complex. A total of 2,999 rsRNAs were identified in this study which exhibited exclusive expression in the presence of DGCR8 ecomplex. Most of them displayed the presence of typical hairpin loop precursor structure.
After the introduction of NGS-sequencing technologies many new miRNAs were reported in miRBase which do not follow the above criteria such as presence of terminal loop region (hsa-miR-181d, hsa-miR-141) absence of pairing mature miRNAs (in about 45% of total mature miRNAs reported (hsa-miR-944, hsa-miR-378d-2), mature miRNAs coming from loop regions instead of stem region (hsa-miR-451a, hsa-miR-7111), rsRNA reads mapping on other region instead of reported known miRNAs (hsa-miR-5680, hsa-miR-5697), mature miRNAs with no overhangs (hsa-miR-7108). These miRNAs suggest that following only the canonical or traditional pathway for miRNA biogenesis could become a bottleneck in addressing a larger question or regulatory sRNAs, creating bias and report mainly those miRNAs which follow the similar characteristics patterns as the already reported ones. The data here provides the list of miRNAs which follow atypical look and deviate from the standard concepts of miRNAs. They are reported in miRBase version 21.
Similarly, a huge number of iso-miRs, phased miRNAs and miRNA offset RNAs (mORs) have been reported recently, suggesting that from a single precursor itself a large number of regulatory sRNAs arise in actual, grossly disobeying typical miRNA properties defined so far. Majority of such a typical miRNAs/rsRNAs display lower abundance or highly spatio-temporal expression, a reason why most of the standard discovery protocols missed them so far or confused them with random degradation product.
Being tagged as random product, most of them have been discarded. Therefore, in the way of discovery of such regulatory sRNAs, a sensible big objective has been to first establish them as non-random elements. To address this all collectively, nclduing the funcational and regulatory importance of such sRNAs, the present study worked with
three fundamental approaches:
1) Recurrence of any given sRNA across large number of experimental samples/individuals provides strong evidence for non-random existence of any given sRNA,
2) For the given samples, if the RNA-seq data or whole transcriptome expression data is available, it is possible to estimate the anti-correlation for expression between a sRNA and its putative targets, multiple times in re-affirmative manner. Use of proteome abundance data in measuring anti-correlation with the target gene immensely supports study of the regulatory impacts of sRNAs on its targets.
3) Support of data from different technologies like AGO-HITS CLIP sequencing and CLASH provide very high confidence experimental proof of interactions between the sRNA and targets.
This many layers of cross validation using experimental data from different sources provide a high confidence support to call a sRNA's existence as non-random element having regulatory impact.
1) Cancer is once of the most dreaded health condtions spreading very fast and yet unanswered.
2) A huge amount of experimental data is available which fulfull the above mentioned three prime requirements to carry out this study. Presence of publicly available data for large number of replicates, individuals and experimental conditions in portals like GEO
and The Cancer Genomics Atlas (TCGA) make it possible to successfully carry a study of this magnitude, providing multilayered evidences.
The Cancer Genomics Atlas (TCGA) in the recent times has emerged as the prime repository for all cancer related genomics data. TCGA hosts an enormous amount of data for approximately 20 various cancer types, freely available for researchers worldwide. The data includes RNA-seq based digital gene expression, sRNA reads, protein based expression, Microarray based gene expression, DNA methylation data etc. The given image illustrates the volume of data and information contributed by TCGA while conducting the present study. The TCGA data used in the present study were taken after TCGA's permission, and the present study abides by their condtions for use.
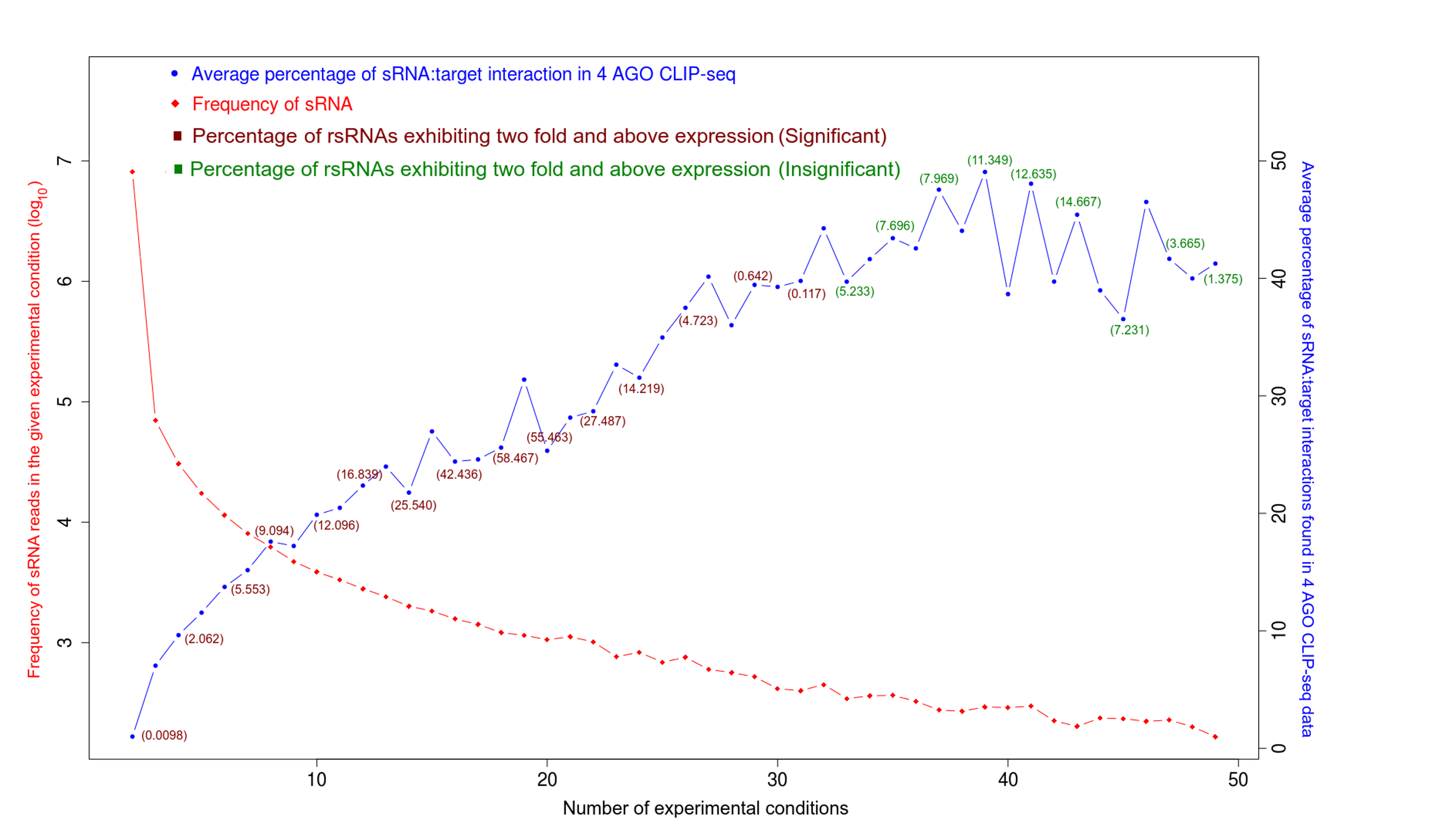
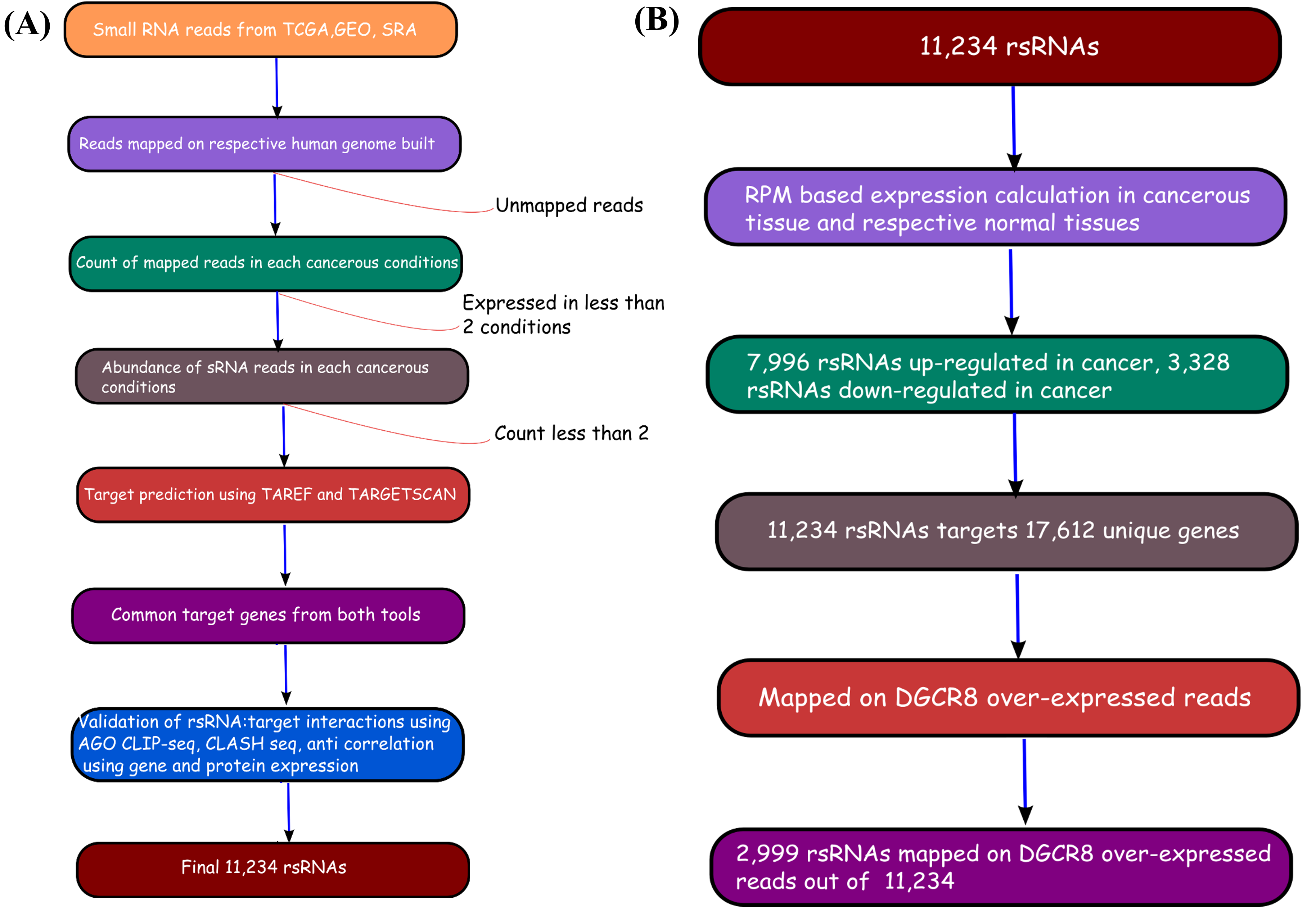
To identify if these DGCR8 specific rsRNAs exhibited pre-miRNA like typical hairpin loop structure, their genomic sequences with 200 bp flanking regions were scanned using MirEval and RNAfold. It was found that 2,204 rsRNAs precursors were having hair-pin loop precursor like structure. 1,125 such sRNAs were present almost perfectly within the stem region.
Validation of rsRNA:target inetaractions was done by searching the inetractions in the Argonaute sequencing data downloaded from starBase version 2. A total of 149,344, 150,049, 145,561 and 148,251 novel putative small regulatory RNA:target interactions were identified for 8,036, 6,247, 8,342 and 7,196 unique rsRNAs and 14,265, 12,448, 14,438 and 13,674 unique targeted genes, for AGO1, AGO2, AGO3 and, AGO4-CLIP sequencing data, respectively.
CLASH-seq data was also used for the identification of rsRNA: target interactions. As compared to CLIP-seq, CLASH-seq data gives more specific information about the interactions as the interactions are precisely arrested through ligation of the target site and targeting small RNA. Using CLASH-seq data, 16,371 unique genes were found being targeted by 10,048 putative rsRNAs, comprising 474,770 unique target interactions. The list of common and unique genes identified in AGO based HITS-CLIP data and CLASH data.
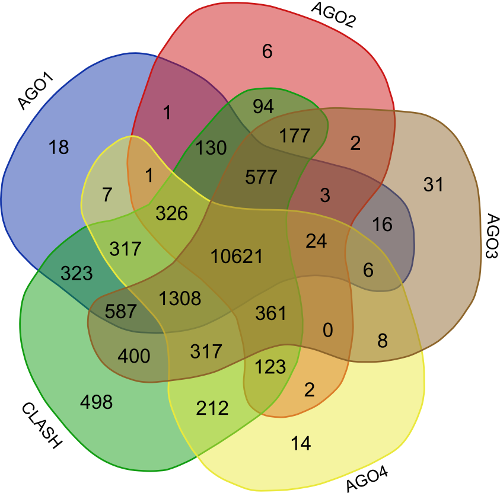
Further to validate the interactions, expression anti-correlation based validation study was performed using sRNA expression (RPM) and expression of target genes (RPKM) obtained from TCGA and GEO, a total of 3,013 cancerous and normal tissue based experimental conditions were considered. For those cancerous conditon in which RPKM data was not available, microarray absed expression date from TCGA (for 137 patients and normal conditions) were used. A high level of agreement was observed between different methods of validation for the identified rsRNA:target interactions.
In order to find the common targets identified reported by the avarious above mentioned validation approaches i.e. (AGO1-4, CLASH-seq, Protein abundance support, RPKM based, Microarray based) a venn representation was done. As apparent here, for most of the interactions two or more methods agreed, strongly suggesting the regulatory existence of these sRNAs.
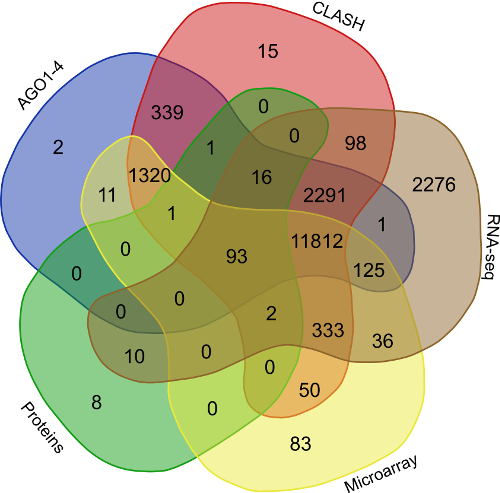
These novel sRNAs were found regulating many genes including some important genes involved in cacer like BRCA2, p53, Rb, Myc, 14-3-3 epsilon, CycD and CycE, and in general pathways related to cancer.
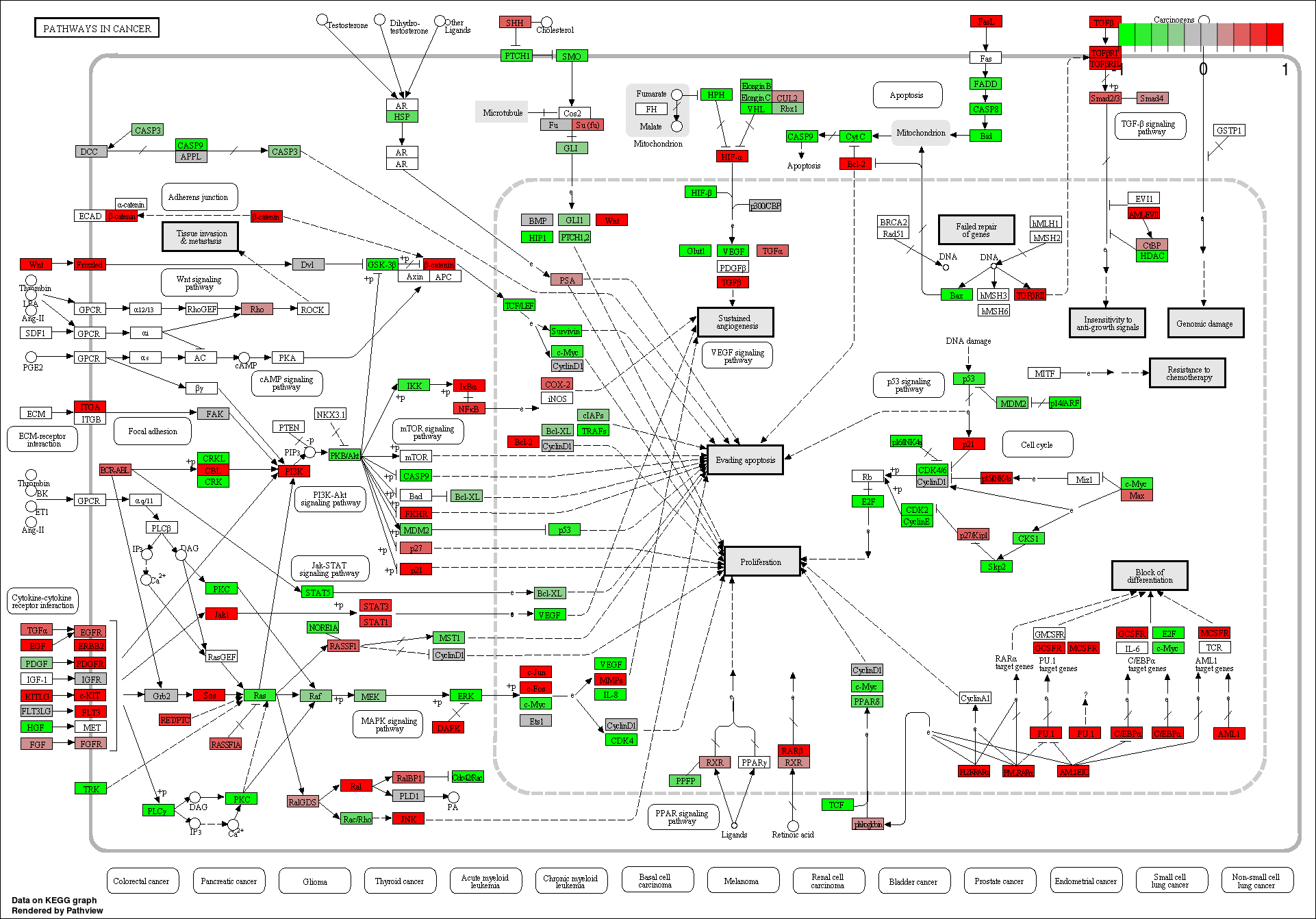
From the mapped data, it was found that these small regulatory RNAs have multiple biogenesis loci mainly belonging to repetitive elements (48.22%), Intronic region (36.02%) and ncRNA (9.31%) regions. Many of the known miRNAs have been reported from intronic region, in-fact 46.03% (866 out of 1881) miRNAs, in current version of miRBase are from the intronic regions.
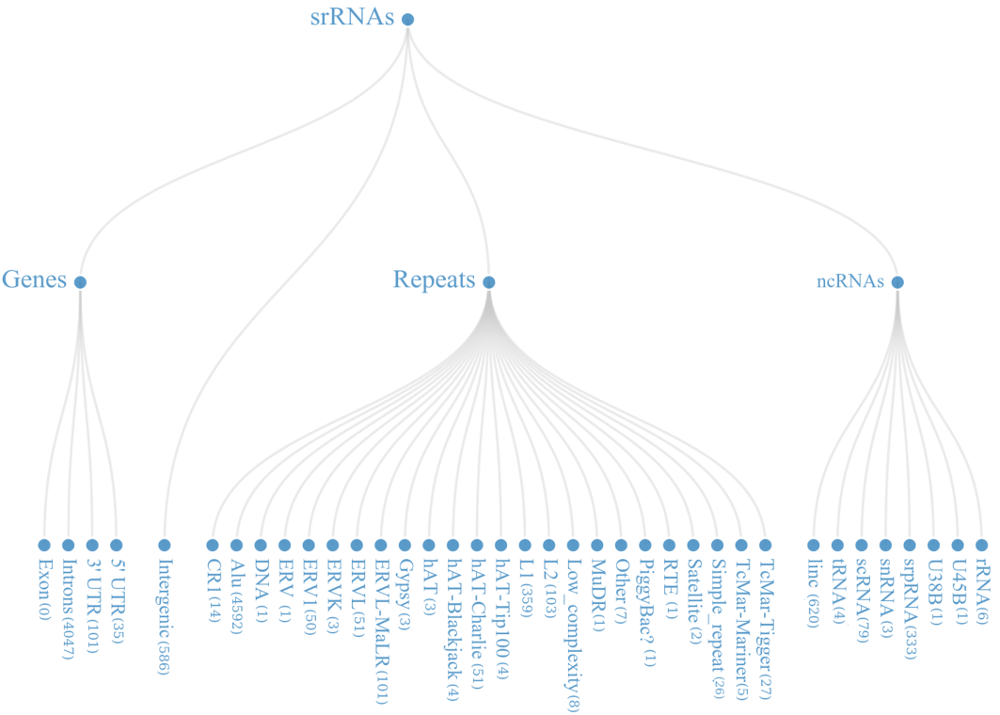
Several rsRNAs were found originating from the Alu elements. While observing the distribution profile of these sRNAs across the length of Alu consensus, it was observed that, these rsRNAs follow a conserved pattern of biogenesis for a number of different experimental conditions. A Multiple chart visualization of Alu derived sRNA expression profile variations for the different cancer states and normal tissues has been made available to showcase this behavior of Alu derived sRNAs . The sRNAs originating from Alu displayed high conservation of profiles across the individuals, which was also different between the normal and cancer samples. A series of t-tests between cancer v/s normal conditions gave consistently significant p-values (p<0.05) for such observation, suggesting the sRNA profile originating from Alu differ significantly between cancer and normal states
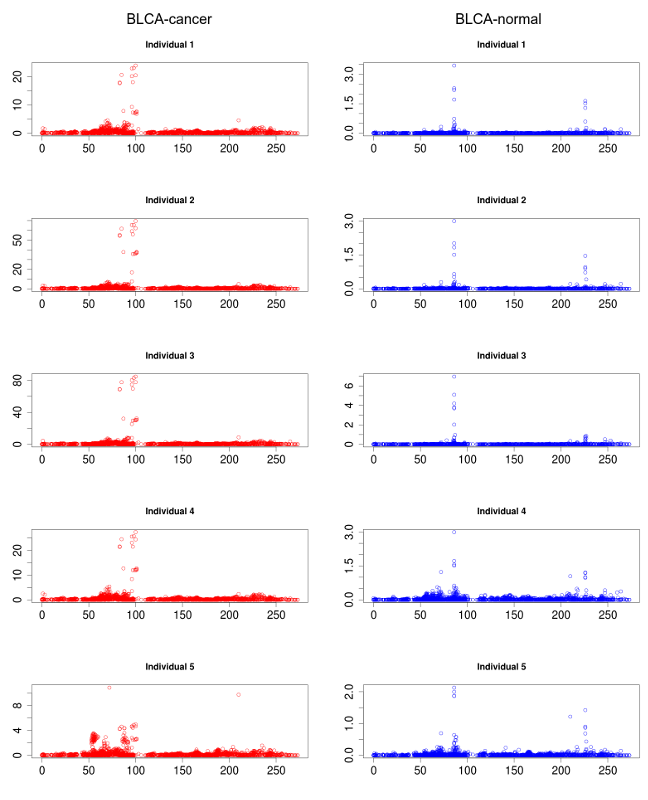
Percentage of reads distribution on rsRNAs originating from repeats were also calculated. This analysis was performed to normalize the rsRNA reads as a given rsRNA from repeats could map across multiple loci. It was found that rsRNAs from ERVL and Alu families were distributed non-randomly across the genome followed by other repeat families.
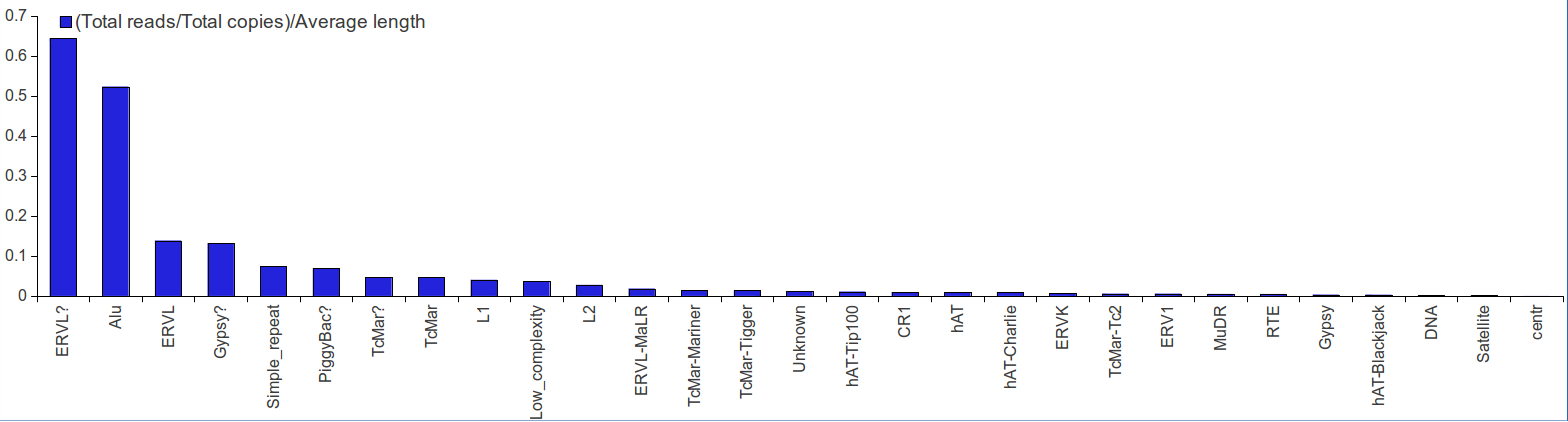
The pathway enrichment analysis of rsRNAs significantly up-regulated in cancer and originating from Alu regions showed that these rsRNAs target genes were involved in regulation of apoptosis, colorectal cancer, renal cell carcinoma, melanoma, small cell lung cancer, pancreatic cancer and chronic myeloid leukemia pathways. Whereas rsRNAs significantly up-regulated in normal conditions target genes involved in apoptosis, neuroactive ligand receptor interaction, colorectal cancer, renal cell carcinoma, fatty acid biosynthesis, tight junction, glycosaminoglycan degradation, cell cycle.
One such novel small regulatory RNA derived from Alu, identified as "rsRNA-6458-n", targets some important genes like ADAR, ATM, BCL2L1, BIRC2, ERBB3, ITGA2, NRAS, RPS6, SRC, STAT3, SYK, VHL and YWHAB. One of its important target, SRC is an important component in signal transduction which is usually found over-expressed in cancer conditions. rsRNA-6458-n was found exhibiting higher expressions (two fold and above) in the normal tissue for READ, KIRC, LUAD, BRCA, HNSC, STAD, PRAD, LUSC, UCEC, OV and BLCA types of cancer states. Consequently, the expression of its target gene, SRC, exhibited over expression in these cancerous states in terms of RNA abundance (RPKM) as well as in terms of protein abundance.
These identified rsRNAs were clustered using four different methods, namely 1) seed region similarity ( comapred with known miRNA seeds) , 2) sRNA length and Argonatue association, 3) All smaller sRNAs covered by a single long sRNA (longest coordinates bound based) and, 4) Expression based clustering. These clusterings were done to identify the rsRNAs sharing similar properties. rsRNAs in a cluster were found targeting same/similar gene, regulating similar pathways and similar functions. Expression based clustering resulted into 362 distinct clusters which includes 7,292 rsRNAs. These rsRNAs shared high co-expression with each other ("r" > 0.5) calculated for 4,997 experimental conditions.
Clusters identified through the given approaches were analyzed for overlap measure among them. High commonalities between the clusters generated based upon different properties suggest a degree of similarity and relationship between the two properties. A 4X4 matrix was generated for similarity scoring between the clusters of above mentioned four types. It was found that expression based clusters shared ~82% similarity with the clusters generated using coordinates bound based clustering, 22% with seed clusters and 0.23% with length based clustering. Coordinates bound based clusters displayed 77% overlap with seed based cluster and 0.09% clusters overlap with length based clustering. This analysis suggested that the three clustering methods based on seed region, coordinates bound and expression similarity agreed with each other, where the co-expressed sRNAs were also similar to each other in terms of genomic coordinates, which also shared a good amount of common targets.
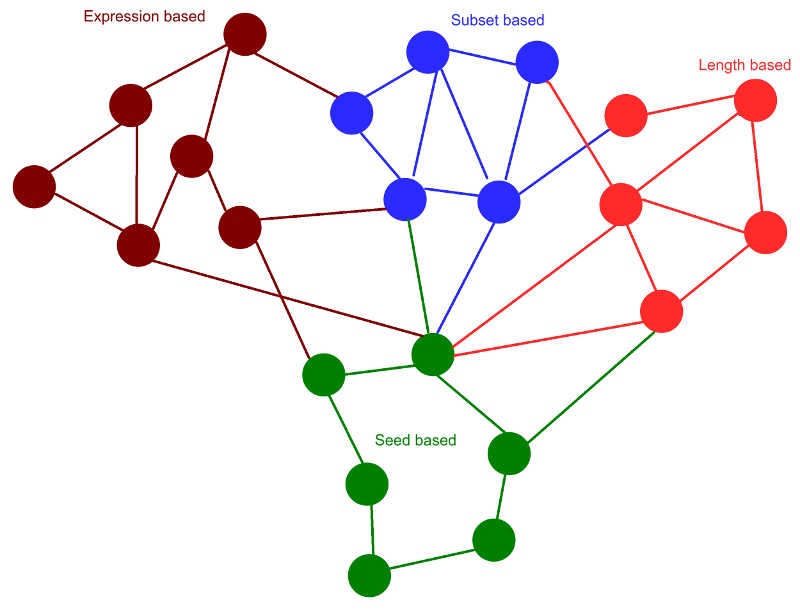
Several novel regulatory small RNAs were found significantly differentially expressed between cancerous and normal conditions. The differential expression was evaluated across large number of individual samples, followed by t-test for significance between normal sample sets and cancer sample sets, for every cancer condition.
Cancer Tissue | rsRNA ID | Fold change in Cancer | P-value | rsRNA ID | Fold change in Normal | P-value |
---|---|---|---|---|---|---|
BLCA | rsRNA-7062-n | 140.36 | 0.02907 | rsRNA-7208-n | 4.39 | 0.01157 |
BLCA | rsRNA-9580-n | 150.21 | 2.69E-013 | rsRNA-6454-n | 4.46 | 0.02114 |
BLCA | rsRNA-1754-n | 154.26 | 5.41E-008 | rsRNA-4562-n | 4.49 | 0.02677 |
BLCA | rsRNA-3397-n | 158.76 | 0.0001006 | rsRNA-9520-n | 4.87 | 0.001836 |
BLCA | rsRNA-11206-n | 162.45 | 2.89E-013 | rsRNA-10291-n | 5 | 0.0241 |
BLCA | rsRNA-1365-n | 166.76 | 1.48E-010 | rsRNA-3722-n | 5.1 | 0.04994 |
BLCA | rsRNA-10684-n | 173.21 | 1.83E-008 | rsRNA-10119-n | 6.59 | 0.01585 |
BLCA | rsRNA-5987-n | 173.43 | 3.17E-012 | rsRNA-9620-n | 6.73 | 0.001029 |
BLCA | rsRNA-813-n | 197.63 | 2.20E-016 | rsRNA-6728-n | 6.97 | 0.01342 |
BLCA | rsRNA-1558-n | 273.63 | 1.69E-011 | rsRNA-7952-n | 7.15 | 0.002217 |
BRCA | rsRNA-11080-n | 50.33 | 2.20E-016 | rsRNA-7343-n | 4.13 | 0.002289 |
BRCA | rsRNA-1017-n | 51.78 | 2.82E-013 | rsRNA-7089-n | 4.16 | 0.002339 |
BRCA | rsRNA-4331-n | 52.93 | 2.20E-016 | rsRNA-7002-n | 4.29 | 0.009076 |
BRCA | rsRNA-2973-n | 55.6 | 2.20E-016 | rsRNA-1335-n | 4.65 | 0.03145 |
BRCA | rsRNA-3808-n | 66.72 | 2.20E-016 | rsRNA-1432-n | 6.4 | 1.05E-011 |
BRCA | rsRNA-2432-n | 86.48 | 3.22E-008 | rsRNA-4362-n | 6.51 | 1.12E-011 |
BRCA | rsRNA-6663-n | 99.52 | 2.73E-014 | rsRNA-9417-n | 6.69 | 0.01212 |
BRCA | rsRNA-7481-n | 111.78 | 2.20E-016 | rsRNA-10829-n | 9.12 | 2.15E-006 |
BRCA | rsRNA-7063-n | 149.74 | 4.63E-016 | rsRNA-7392-n | 13.43 | 0.006228 |
BRCA | rsRNA-10204-n | 194.9 | 2.24E-013 | rsRNA-10410-n | 18.73 | 0.03788 |
COAD | rsRNA-4606-n | 57.01 | 2.20E-016 | rsRNA-7553-n | 16.02 | 0.01836 |
COAD | rsRNA-7906-n | 58.99 | 0.02553 | rsRNA-5491-n | 16.26 | 0.007327 |
COAD | rsRNA-3808-n | 62.24 | 1.85E-012 | rsRNA-8900-n | 17.16 | 0.003794 |
COAD | rsRNA-2973-n | 67.37 | 2.81E-008 | rsRNA-8708-n | 18.47 | 0.006546 |
COAD | rsRNA-11212-n | 78.37 | 2.28E-006 | rsRNA-7914-n | 18.5 | 0.01926 |
COAD | rsRNA-11080-n | 80.38 | 2.93E-012 | rsRNA-11171-n | 18.88 | 0.02686 |
COAD | rsRNA-7063-n | 81.23 | 1.47E-011 | rsRNA-7392-n | 19.95 | 0.01256 |
COAD | rsRNA-3599-n | 94 | 6.73E-008 | rsRNA-219-n | 27.05 | 0.01528 |
COAD | rsRNA-5004-n | 124.33 | 5.54E-005 | rsRNA-4125-n | 27.41 | 0.04796 |
COAD | rsRNA-4146-n | 542.44 | 4.43E-006 | rsRNA-359-n | 90.28 | 0.00621 |
HNSC | rsRNA-3940-n | 23.76 | 2.20E-016 | rsRNA-4260-n | 4.38 | 1.63E-005 |
HNSC | rsRNA-7039-n | 23.92 | 0.008792 | rsRNA-7076-n | 4.5 | 0.0009993 |
HNSC | rsRNA-11210-n | 24.59 | 1.82E-014 | rsRNA-6973-n | 4.54 | 2.20E-016 |
HNSC | rsRNA-2088-n | 24.94 | 4.13E-013 | rsRNA-2227-n | 5.38 | 0.01776 |
HNSC | rsRNA-1597-n | 25.73 | 3.58E-011 | rsRNA-9083-n | 5.78 | 0.02459 |
HNSC | rsRNA-6870-n | 27.15 | 0.003742 | rsRNA-4387-n | 7.82 | 0.0001261 |
HNSC | rsRNA-5403-n | 28.49 | 1.67E-005 | rsRNA-1828-n | 8.88 | 1.99E-009 |
HNSC | rsRNA-9553-n | 32.22 | 1.05E-008 | rsRNA-2634-n | 11.22 | 0.04651 |
HNSC | rsRNA-9270-n | 46.36 | 1.11E-009 | rsRNA-8264-n | 12.56 | 0.03923 |
HNSC | rsRNA-5975-n | 47.33 | 3.04E-008 | rsRNA-5483-n | 14.93 | 0.0009894 |
KIRC | rsRNA-7481-n | 30.42 | 0.01488 | rsRNA-9299-n | 3.58 | 3.70E-005 |
KIRC | rsRNA-3599-n | 32.5 | 0.0003393 | rsRNA-5530-n | 3.63 | 0.01643 |
KIRC | rsRNA-6093-n | 35.82 | 0.0001098 | rsRNA-7697-n | 4.11 | 5.56E-005 |
KIRC | rsRNA-2025-n | 41.17 | 1.59E-014 | rsRNA-3539-n | 4.2 | 1.06E-012 |
KIRC | rsRNA-6503-n | 41.53 | 2.40E-011 | rsRNA-7730-n | 4.21 | 5.97E-006 |
KIRC | rsRNA-9173-n | 42.48 | 0.01697 | rsRNA-2277-n | 4.23 | 2.93E-010 |
KIRC | rsRNA-10131-n | 44.01 | 1.51E-008 | rsRNA-8251-n | 4.26 | 3.97E-006 |
KIRC | rsRNA-1194-n | 50.23 | 0.004899 | rsRNA-4976-n | 4.32 | 3.91E-014 |
KIRC | rsRNA-4146-n | 75.19 | 4.05E-011 | rsRNA-3441-n | 4.5 | 0.0002262 |
KIRC | rsRNA-8879-n | 98.17 | 2.20E-016 | rsRNA-2373-n | 6.28 | 0.005737 |
KIRP | rsRNA-10016-n | 17.98 | 0.008155 | rsRNA-7908-n | 10.95 | 0.004548 |
KIRP | rsRNA-9563-n | 22.79 | 0.01748 | rsRNA-1936-n | 12.13 | 0.02918 |
KIRP | rsRNA-9290-n | 24.16 | 0.01314 | rsRNA-8859-n | 13.14 | 0.04315 |
KIRP | rsRNA-2973-n | 25.62 | 0.005962 | rsRNA-1498-n | 13.31 | 0.003261 |
KIRP | rsRNA-7295-n | 27.75 | 0.02244 | rsRNA-4772-n | 16.93 | 0.03947 |
KIRP | rsRNA-9338-n | 28.62 | 0.02839 | rsRNA-10677-n | 19.57 | 0.04369 |
KIRP | rsRNA-1314-n | 31.86 | 0.0001441 | rsRNA-7156-n | 19.57 | 0.01189 |
KIRP | rsRNA-7442-n | 34.45 | 0.02777 | rsRNA-2393-n | 31.8 | 0.03626 |
KIRP | rsRNA-9173-n | 43.58 | 0.03868 | rsRNA-8092-n | 36.95 | 0.002172 |
KIRP | rsRNA-4950-n | 46.67 | 0.01188 | rsRNA-3441-n | 106.05 | 0.03241 |
LUAD | rsRNA-8879-n | 32.16 | 0.0001573 | rsRNA-9213-n | 8.56 | 0.0002941 |
LUAD | rsRNA-8074-n | 32.36 | 2.52E-006 | rsRNA-9520-n | 9.31 | 1.87E-008 |
LUAD | rsRNA-2973-n | 32.36 | 0.01857 | rsRNA-10829-n | 9.31 | 0.0001279 |
LUAD | rsRNA-7658-n | 32.49 | 8.86E-015 | rsRNA-9808-n | 10.4 | 0.006902 |
LUAD | rsRNA-5004-n | 32.66 | 5.86E-005 | rsRNA-5929-n | 12.77 | 1.11E-007 |
LUAD | rsRNA-6911-n | 34.43 | 0.001641 | rsRNA-7952-n | 13.02 | 0.02594 |
LUAD | rsRNA-4982-n | 38.54 | 4.39E-014 | rsRNA-465-n | 16.07 | 0.01844 |
LUAD | rsRNA-6636-n | 52.89 | 2.29E-005 | rsRNA-9221-n | 18.06 | 0.01585 |
LUAD | rsRNA-10576-n | 92.43 | 0.00468 | rsRNA-7392-n | 23.46 | 0.0138 |
LUAD | rsRNA-11080-n | 111.01 | 3.84E-005 | rsRNA-10410-n | 24.26 | 0.000788 |
LUSC | rsRNA-3599-n | 56.15 | 2.86E-007 | rsRNA-4582-n | 7.07 | 0.01295 |
LUSC | rsRNA-6496-n | 58.43 | 0.001655 | rsRNA-10667-n | 7.55 | 3.69E-005 |
LUSC | rsRNA-9256-n | 63.42 | 0.003116 | rsRNA-6091-n | 7.59 | 0.0001526 |
LUSC | rsRNA-1933-n | 65.21 | 0.001441 | rsRNA-5420-n | 7.59 | 7.85E-005 |
LUSC | rsRNA-2567-n | 66.18 | 2.10E-011 | rsRNA-7460-n | 8.2 | 0.002286 |
LUSC | rsRNA-2248-n | 82.53 | 1.62E-010 | rsRNA-6820-n | 8.63 | 1.37E-005 |
LUSC | rsRNA-3257-n | 90.3 | 2.20E-016 | rsRNA-931-n | 10.05 | 1.12E-006 |
LUSC | rsRNA-4436-n | 101.56 | 1.04E-008 | rsRNA-6167-n | 12.82 | 0.007293 |
LUSC | rsRNA-9881-n | 128.07 | 2.20E-016 | rsRNA-5929-n | 13.12 | 0.0003293 |
LUSC | rsRNA-4146-n | 428.84 | 8.12E-010 | rsRNA-10410-n | 27.75 | 0.02072 |
OV | rsRNA-680-n | 39.2 | 2.20E-016 | rsRNA-6136-n | 14.55 | 2.20E-016 |
OV | rsRNA-3032-n | 39.7 | 4.80E-007 | rsRNA-10363-n | 16.2 | 7.21E-012 |
OV | rsRNA-5577-n | 41.6 | 2.20E-016 | rsRNA-1828-n | 16.99 | 2.20E-016 |
OV | rsRNA-10199-n | 44.79 | 2.20E-016 | rsRNA-10791-n | 17.63 | 2.30E-009 |
OV | rsRNA-2242-n | 44.87 | 1.27E-010 | rsRNA-6488-n | 18.07 | 0.01842 |
OV | rsRNA-7462-n | 48.34 | 2.20E-016 | rsRNA-9620-n | 23.16 | 0.02536 |
OV | rsRNA-10402-n | 52.23 | 0.0001216 | rsRNA-11000-n | 27 | 1.79E-008 |
OV | rsRNA-2049-n | 54.18 | 4.82E-007 | rsRNA-3411-n | 39.54 | 6.12E-010 |
OV | rsRNA-1986-n | 56.05 | 0.0003903 | rsRNA-4441-n | 71.63 | 2.20E-016 |
OV | rsRNA-4673-n | 165.65 | 2.47E-012 | rsRNA-7460-n | 114.02 | 2.20E-016 |
PRAD | rsRNA-1271-n | 35.13 | 2.07E-010 | rsRNA-7776-n | 4.54 | 0.04095 |
PRAD | rsRNA-8942-n | 35.44 | 0.0004146 | rsRNA-2606-n | 4.55 | 0.02798 |
PRAD | rsRNA-5993-n | 43.06 | 0.0001654 | rsRNA-1213-n | 4.75 | 7.61E-005 |
PRAD | rsRNA-5049-n | 44.13 | 1.38E-009 | rsRNA-10955-n | 4.82 | 0.003896 |
PRAD | rsRNA-9923-n | 45.92 | 6.54E-009 | rsRNA-8775-n | 4.99 | 9.98E-007 |
PRAD | rsRNA-6355-n | 47.87 | 7.34E-007 | rsRNA-5339-n | 5.01 | 3.11E-005 |
PRAD | rsRNA-1472-n | 54.62 | 0.001062 | rsRNA-10785-n | 5.15 | 0.001983 |
PRAD | rsRNA-4860-n | 59.62 | 0.000138 | rsRNA-1241-n | 5.57 | 0.01212 |
PRAD | rsRNA-4312-n | 61.95 | 0.00755 | rsRNA-7734-n | 6.05 | 4.84E-005 |
PRAD | rsRNA-4994-n | 67.97 | 6.08E-007 | rsRNA-10981-n | 8.71 | 0.004934 |
READ | rsRNA-11037-n | 169.78 | 0.03641 | rsRNA-4287-n | 2.14 | 0.007182 |
READ | rsRNA-5568-n | 171.62 | 0.03825 | rsRNA-10044-n | 2.2 | 0.03419 |
READ | rsRNA-2875-n | 185.68 | 0.01036 | rsRNA-6100-n | 2.45 | 0.03117 |
READ | rsRNA-1547-n | 186.54 | 8.86E-010 | rsRNA-8297-n | 2.46 | 0.03083 |
READ | rsRNA-2218-n | 186.87 | 1.66E-007 | rsRNA-5667-n | 2.6 | 0.01226 |
READ | rsRNA-5026-n | 234.79 | 0.0001037 | rsRNA-10803-n | 3.09 | 2.20E-016 |
READ | rsRNA-8208-n | 521.29 | 3.00E-005 | rsRNA-5763-n | 3.26 | 0.02535 |
READ | rsRNA-7063-n | 1413.02 | 1.87E-005 | rsRNA-10160-n | 3.41 | 0.02195 |
READ | rsRNA-3599-n | 1596.56 | 1.46E-008 | rsRNA-2425-n | 5.47 | 0.02266 |
READ | rsRNA-5004-n | 6239.69 | 9.57E-006 | rsRNA-2373-n | 7.45 | 0.0003594 |
STAD | rsRNA-5005-n | 39.81 | 1.43E-008 | rsRNA-10119-n | 3.69 | 0.00414 |
STAD | rsRNA-8074-n | 42.81 | 7.24E-011 | rsRNA-10046-n | 3.79 | 1.00E-005 |
STAD | rsRNA-2894-n | 43.48 | 1.52E-014 | rsRNA-11185-n | 3.97 | 0.003068 |
STAD | rsRNA-11065-n | 43.78 | 0.01523 | rsRNA-9097-n | 3.99 | 0.006557 |
STAD | rsRNA-4365-n | 47.92 | 0.002176 | rsRNA-9933-n | 4.22 | 2.92E-005 |
STAD | rsRNA-1933-n | 47.92 | 2.55E-009 | rsRNA-10141-n | 4.84 | 0.03617 |
STAD | rsRNA-7799-n | 54.25 | 5.03E-014 | rsRNA-8378-n | 4.86 | 0.01982 |
STAD | rsRNA-5024-n | 72.39 | 6.55E-008 | rsRNA-8395-n | 5.61 | 0.01321 |
STAD | rsRNA-2992-n | 74.17 | 0.025 | rsRNA-6220-n | 6.29 | 0.02675 |
STAD | rsRNA-7223-n | 126.48 | 1.73E-008 | rsRNA-7220-n | 10.71 | 0.03923 |
THCA | rsRNA-3441-n | 4.43 | 3.97E-005 | rsRNA-7940-n | 15.22 | 1.56E-008 |
THCA | rsRNA-7906-n | 4.68 | 2.20E-016 | rsRNA-4301-n | 15.63 | 0.0009558 |
THCA | rsRNA-16-n | 5.56 | 0.0006849 | rsRNA-4853-n | 15.86 | 1.04E-005 |
THCA | rsRNA-3257-n | 5.69 | 3.28E-015 | rsRNA-8870-n | 18.27 | 9.59E-005 |
THCA | rsRNA-8260-n | 5.82 | 0.003016 | rsRNA-8771-n | 19.17 | 1.81E-007 |
THCA | rsRNA-7439-n | 5.86 | 0.005565 | rsRNA-8345-n | 19.19 | 0.0006329 |
THCA | rsRNA-9128-n | 5.91 | 2.20E-016 | rsRNA-2791-n | 19.44 | 0.0004284 |
THCA | rsRNA-6636-n | 6.05 | 5.93E-010 | rsRNA-6997-n | 21.21 | 0.004805 |
THCA | rsRNA-4686-n | 6.82 | 0.0002256 | rsRNA-10793-n | 23.07 | 2.49E-005 |
THCA | rsRNA-6554-n | 7.12 | 2.20E-016 | rsRNA-1832-n | 24.17 | 3.53E-007 |
UCEC | rsRNA-3808-n | 116.17 | 2.20E-016 | rsRNA-9097-n | 6.03 | 0.001132 |
UCEC | rsRNA-4146-n | 117.51 | 5.48E-007 | rsRNA-5387-n | 6.29 | 0.0002645 |
UCEC | rsRNA-7063-n | 124.16 | 2.20E-016 | rsRNA-10667-n | 6.53 | 0.0394 |
UCEC | rsRNA-5915-n | 127.42 | 4.49E-006 | rsRNA-1643-n | 6.81 | 0.0006566 |
UCEC | rsRNA-3599-n | 130.03 | 2.20E-016 | rsRNA-10762-n | 7.54 | 0.001034 |
UCEC | rsRNA-2338-n | 131.17 | 6.81E-009 | rsRNA-4582-n | 8.62 | 0.009029 |
UCEC | rsRNA-10336-n | 142.71 | 2.20E-016 | rsRNA-11156-n | 8.93 | 2.29E-005 |
UCEC | rsRNA-2973-n | 154.87 | 2.20E-016 | rsRNA-8492-n | 8.97 | 0.00245 |
UCEC | rsRNA-5080-n | 160.3 | 0.006781 | rsRNA-9409-n | 10.02 | 0.02379 |
UCEC | rsRNA-11080-n | 217.15 | 2.81E-005 | rsRNA-6820-n | 12.74 | 0.001836 |
ACC | rsRNA-8649-n | 11.84 | 3.46E-005 | rsRNA-7634-n | 3.19 | 0.01726 |
ACC | rsRNA-6573-n | 11.98 | 0.004794 | rsRNA-7899-n | 3.21 | 0.007634 |
ACC | rsRNA-10083-n | 12.4 | 5.39E-006 | rsRNA-7260-n | 3.39 | 0.03738 |
ACC | rsRNA-5594-n | 12.77 | 0.0002168 | rsRNA-3001-n | 3.44 | 0.03262 |
ACC | rsRNA-358-n | 13.37 | 2.26E-005 | rsRNA-10644-n | 3.45 | 0.0155 |
ACC | rsRNA-6964-n | 13.73 | 0.0002076 | rsRNA-5314-n | 3.47 | 0.01572 |
ACC | rsRNA-6931-n | 13.89 | 1.81E-006 | rsRNA-5489-n | 3.61 | 0.01631 |
ACC | rsRNA-9130-n | 27.48 | 0.0005026 | rsRNA-4189-n | 4.38 | 0.02726 |
ACC | rsRNA-7124-n | 31.06 | 3.15E-005 | rsRNA-9215-n | 4.68 | 0.01465 |
ACC | rsRNA-8849-n | 53.78 | 2.20E-005 | rsRNA-11083-n | 8.73 | 0.01585 |
DCIS | rsRNA-4485-n | 9.84 | 0.01172 | rsRNA-8332-n | 14.8 | 0.01278 |
DCIS | rsRNA-1888-n | 9.97 | 0.03678 | rsRNA-5670-n | 16.45 | 0.005579 |
DCIS | rsRNA-529-n | 10.09 | 0.02255 | rsRNA-4691-n | 21.07 | 0.003245 |
DCIS | rsRNA-6922-n | 10.09 | 0.04589 | rsRNA-7660-n | 21.1 | 0.02702 |
DCIS | rsRNA-6557-n | 15.14 | 0.002119 | rsRNA-1648-n | 27.91 | 0.04295 |
DCIS | rsRNA-4216-n | 20.65 | 0.01928 | rsRNA-5075-n | 29.09 | 0.0005523 |
DCIS | rsRNA-548-n | 33.69 | 0.0006843 | rsRNA-10570-n | 29.22 | 0.0007474 |
DCIS | rsRNA-2959-n | 40.1 | 0.04197 | rsRNA-11092-n | 45.05 | 0.03561 |
DCIS | rsRNA-8027-n | 73.68 | 0.01164 | rsRNA-8294-n | 45.76 | 0.0002412 |
DCIS | rsRNA-10368-n | 110.24 | 0.009909 | rsRNA-5909-n | 46.29 | 0.003855 |
IDC | rsRNA-361-n | 10.36 | 0.01004 | rsRNA-8294-n | 5.4 | 0.0009205 |
IDC | rsRNA-1834-n | 10.62 | 0.03809 | rsRNA-4691-n | 5.46 | 0.008105 |
IDC | rsRNA-221-n | 10.87 | 0.02676 | rsRNA-5452-n | 5.48 | 0.009395 |
IDC | rsRNA-402-n | 11.34 | 0.001479 | rsRNA-1339-n | 5.67 | 0.04641 |
IDC | rsRNA-10130-n | 12.23 | 0.00935 | rsRNA-161-n | 6.17 | 0.03443 |
IDC | rsRNA-1314-n | 13.07 | 0.01647 | rsRNA-8332-n | 8.63 | 0.0164 |
IDC | rsRNA-2957-n | 13.67 | 0.04502 | rsRNA-6812-n | 9.39 | 0.02839 |
IDC | rsRNA-2959-n | 21.67 | 0.002465 | rsRNA-10570-n | 14.99 | 0.0009916 |
IDC | rsRNA-2035-n | 22.27 | 0.002806 | rsRNA-5475-n | 21.62 | 0.001151 |
IDC | rsRNA-10368-n | 55.96 | 0.01004 | rsRNA-7660-n | 45.84 | 0.02369 |
KIDNEY | rsRNA-11006-n | 5.01 | 0.04456 | rsRNA-2277-n | 4.21 | 0.03087 |
KIDNEY | rsRNA-7688-n | 5.02 | 0.0005859 | rsRNA-10624-n | 4.42 | 0.04426 |
KIDNEY | rsRNA-3443-n | 5.14 | 0.03747 | rsRNA-7076-n | 4.69 | 0.008871 |
KIDNEY | rsRNA-8324-n | 5.33 | 0.03102 | rsRNA-9379-n | 5.07 | 0.01436 |
KIDNEY | rsRNA-5074-n | 5.49 | 0.04186 | rsRNA-10323-n | 5.2 | 0.0452 |
KIDNEY | rsRNA-9061-n | 5.7 | 0.02896 | rsRNA-6454-n | 6.68 | 0.04664 |
KIDNEY | rsRNA-7984-n | 6.44 | 0.01275 | rsRNA-4463-n | 7.12 | 0.007212 |
KIDNEY | rsRNA-5790-n | 7.46 | 0.03852 | rsRNA-7782-n | 9.64 | 0.02606 |
KIDNEY | rsRNA-11232-n | 11.81 | 0.01847 | rsRNA-4009-n | 10.42 | 0.01599 |
KIDNEY | rsRNA-10243-n | 12.38 | 0.004851 | rsRNA-6084-n | 16.11 | 0.03772 |
Pathway enrichment analysis of the rsRNAs up-regulated in cancer shows that the rsRNAs target genes were enriched for apoptosis, renal cell carcinoma, role of brca1 brca2 and atr in cancer susceptibility, caspase cascade in apoptosis and EGFR1 Signaling Pathway(Mus musculus). Whereas, rsRNAs up-regulated in normal tissues were found targeting genes involved in induction of apoptosis through dr3 and dr4/5 death receptors, vegf hypoxia and angiogenesis, hedgehog signaling pathway, jak stat signaling pathway and Signaling Pathways in Glioblastoma(Homo sapiens).
An interesting case is rsRNA 9881 . This small regulatory RNA was found overexpressed in almost all cancer states studied here, suggesting about some central points being affected by this regulatory small RNA. There were 20 different target genes which were found strongly negatively correlated to its expression. A closer analysis revealed that the target genes were enriched for pathways critical for cell development and cancer, at the interfaces of diverse pathways (apoptosis, cell death, p53 signaling, hiv-1 nef, caspase cascade, TLR, TNFR-1 signaling and FAS pathway), reasoning why the regulatory small RNA 9881 was found abundant in most of the studied cancer conditions. The figure below shows how its target genes are interlinked and positioned, making it critical for cancer conditions. Adiopogenesis was the common factor found most affected.
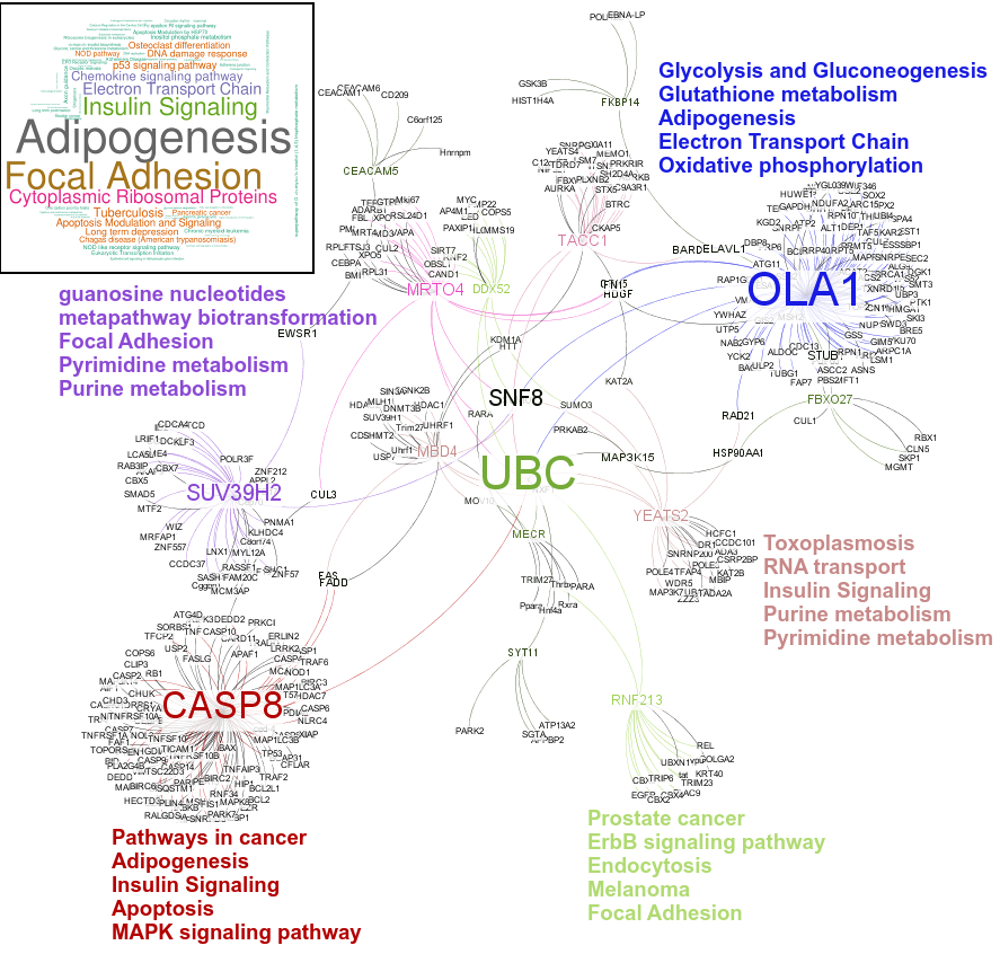
These novel rsRNAs (11,234 in this study) were found to regulate many important biological pathways. Related to cell growth and development and pathways which decide the fate of the cell converting from normal to tumor cell. Some of the pathways include pathways in cancer (hsa05200); Apoptosis (hsa04210) ; Cell cycle (hsa04110); p53 signaling pathway (hsa04115) and many more important pathways.
Browse rsRNAs
Sequence Search
small RNAs data for which AGO/CLASH support was not found
List of small RNAs which was not supported by interaction sequencing data.Please find list here
Browse results by
TCGA barcode Details
Supplementary Details
Contact us
Studio of Computational Biology & Bioinformatics,
Biotech Division,
CSIR-Institute of Himalayan Bioresource Technology,
Palampur 176061 (Himachal Pradesh), India
Email:ravish@ihbt.res.in
Website:http://scbb.ihbt.res.in/